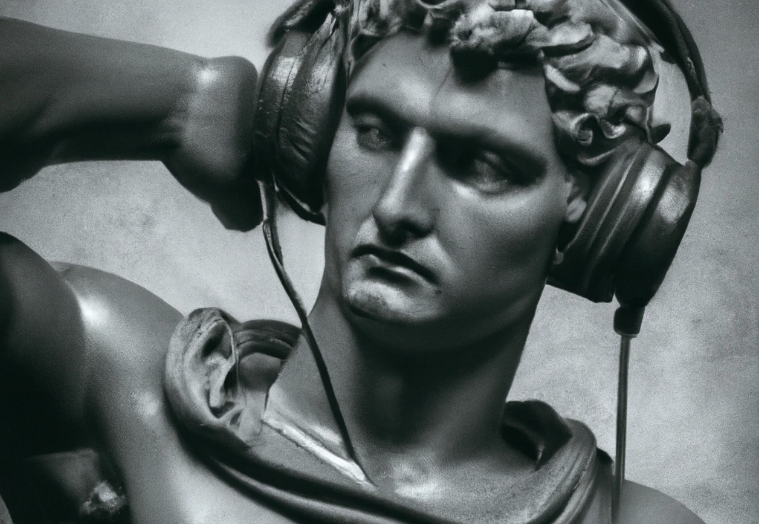
Generative AI refers to a class of artificial intelligence systems that are able to generate new and unique outputs, such as images, text, or music. These systems are based on deep learning algorithms, such as generative adversarial networks (GANs) and variational autoencoders (VAEs), which can learn a given dataset’s underlying patterns and distributions.
Generative AI Advantages
1) New and Unique Outputs
One of the key advantages of generative AI is its ability to create new and unique outputs. For example, a generative AI system trained on a dataset of images of faces can be used to generate new, never-before-seen images of faces. This has many potential applications, such as in the field of computer graphics and animation, where it could be used to create realistic and detailed images of characters or environments.
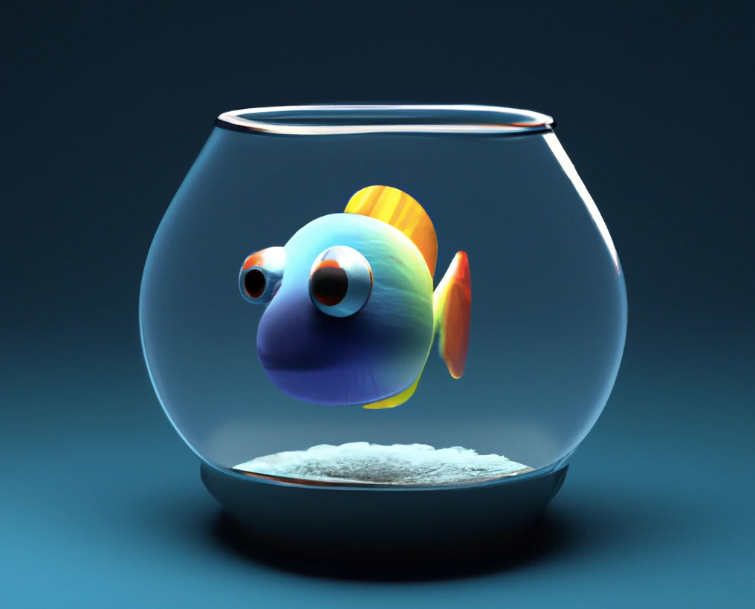
Advantages of Generative AI in Art
With the ability to generate unique and original pieces, Generative AI models have become invaluable tools for artists and content creators alike. In the realm of art, generative AI allows artists to explore new artistic styles, experiment with different forms and techniques, and even collaborate with the machine to create stunning and thought-provoking works. The technology can generate paintings, sculptures, and digital art pieces, providing endless inspiration and pushing artists to explore uncharted territories.
Advantages of Generative AI in Content Creation
In the realm of content creation, generative AI has opened up a world of possibilities. From writing compelling articles and blog posts to generating social media captions and advertising copy, AI models can assist creators in generating engaging and tailored content for their audience. This not only saves time and effort but also ensures a steady stream of fresh and relevant content. Additionally, generative AI can aid in creating music, designing logos and graphics, and even generating immersive virtual reality experiences, expanding the creative horizons of content creators across various industries.
Advantages of Generative AI in Storytelling
One of the most exciting applications of generative AI is in storytelling. AI models can generate narratives, characters, and even entire storylines, providing a wellspring of inspiration for writers and filmmakers. These models can analyze vast amounts of existing literature and media, learning the patterns and structures of storytelling, and then generate new narratives that adhere to these principles while introducing novel and unexpected elements. This opens up new avenues for storytelling, blurring the lines between human and machine creativity, and challenging traditional notions of authorship.
Generative AI has unlocked exciting possibilities in the realms of images and videos. Its manipulation and transformative capabilities offer new avenues for artistic expression, content creation, and immersive storytelling. As this technology continues to evolve, it is essential to leverage its power responsibly and ensure its positive impact on society.
Mohith Agadi, Founder at Fact Protocol
While generative AI has undeniably brought about transformative advancements in art, content creation, and storytelling, it also raises ethical questions and prompts discussions about the role of human creativity. As AI continues to evolve, striking a delicate balance between human ingenuity and machine assistance becomes crucial. Nonetheless, generative AI offers a powerful set of tools that augment human creativity, enabling artists, content creators, and storytellers to explore uncharted territories and push the boundaries of their respective fields.
2) Ability to Learn
Another advantage of generative AI is its ability to learn the underlying patterns and distributions of a dataset. This allows it to generate outputs that are similar to, but not identical to, the input data. This can be used for a variety of tasks, such as image and video synthesis, text generation, and music composition.
3) Data Augmentation
Generative AI can also be used for data augmentation, a technique where the model creates new data from the given data. This can be used to increase the amount of data available for training a machine learning model, which can improve its performance.
Generative AI Disadvantages
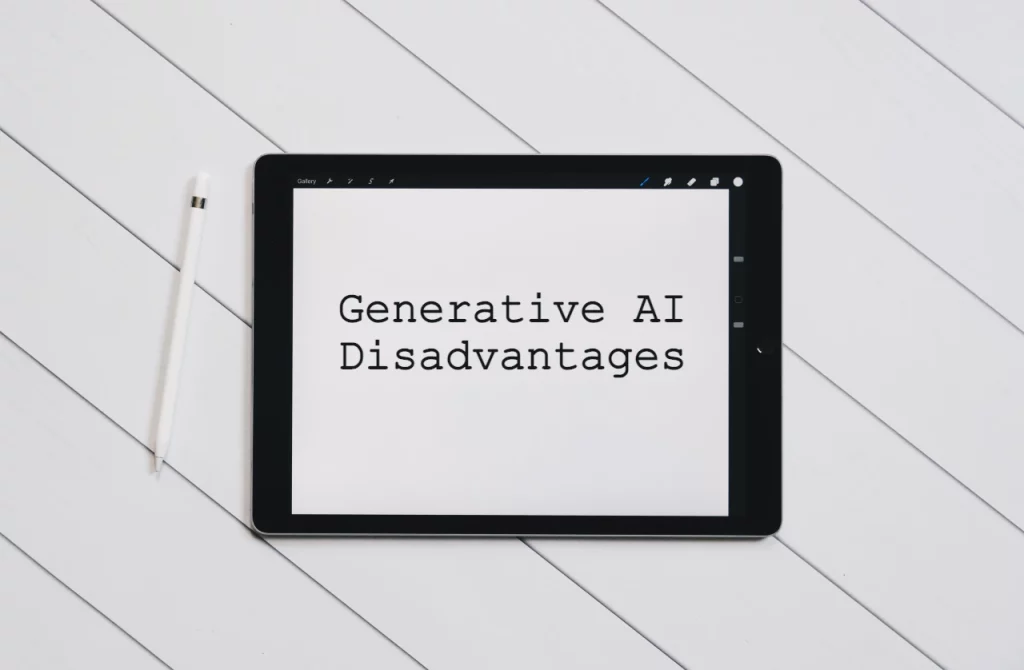
Generative AI, like any technology, has its disadvantages. One of the main concerns with generative AI is the potential for bias in the training data, which can lead to biased or discriminatory outcomes. Another issue is the lack of control over the generated outputs, which can result in unethical or inappropriate content. Additionally, generative AI models can be computationally expensive and require large amounts of training data, which can be a barrier to entry for smaller companies or individuals.
Let’s explore these disadvantages one by one:
1) Limited creativity
While generative AI can create new data based on existing patterns, it is limited in terms of creativity and originality. It can only generate new data based on what it has learned from existing data and cannot think beyond that.
2) Bias
Generative AI can also be biased if the data it was trained on is biased. For example, if the data used to train a generative AI model is biased against a particular group of people, the generated data may also reflect that bias.
3) Limited Application
Generative AI is best suited for applications where there is a large amount of existing data to train on. In cases where there is limited data available, or the data is highly complex, generative AI may not be effective.
4) Resource-intensive
Generative AI requires significant computing resources and training time. It can be expensive to train and deploy generative AI models, which may limit its widespread adoption.
5) Ethical Concerns
Generative AI can be used for malicious purposes, such as generating fake news, deepfakes, or other types of false information. This raises ethical concerns about the potential misuse of the technology.
Generative AI Limitations and Challenges
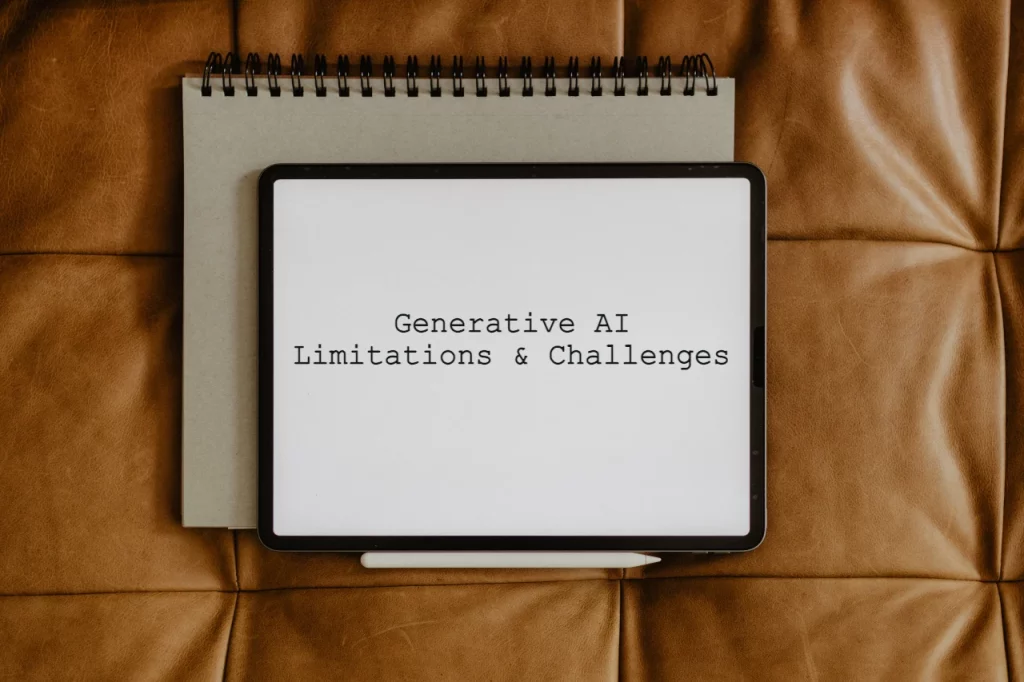
Generative AI also has several limitations and challenges. While some of these may overlap with the contents we discussed in disadvantages, some are not:
1) Quality of Generated Outputs
Generative AI systems may not always produce high-quality outputs, and the generated outputs may contain errors or artifacts. This can be due to a variety of factors, such as a lack of data, poor training, or an overly complex model.
2) Control Over the Generated Outputs
Generative AI systems are typically trained on a dataset and can generate new outputs that are similar to, but not identical to, the input data. However, it can be difficult to control the specific characteristics of the generated outputs.
3) Computational Requirements
Generative AI systems typically require large amounts of data and computational resources to train. This can be expensive and time-consuming, which can be a barrier to entry for some organizations.
4) Bias and Fairness
Generative AI systems can inadvertently replicate biases present in the training data. This can lead to unfair or discriminatory results, particularly if the training data contains biased information.
5) Explainability and Interpretability
Generative AI models can be complex and opaque, making it difficult to understand how they are making their predictions. This can be a challenge when trying to ensure that the model is making fair and unbiased decisions.
6) Safety and Security
Generative AI systems can be used to generate realistic and convincing fake images, videos, and text, which can be used to spread misinformation or propaganda. This highlights the importance of developing safety and security measures to prevent the malicious use of generative AI.
Addressing Limitations and Challenges
There are several ways to address the limitations and challenges of generative AI. Remember that it may not be very wise to think that we can address all the challenges 100% efficiently, because we are still early to this new tech in town. So, let’s explore them one by one in the same order we discussed above:
Quality of Generated Outputs: To improve the quality of generated outputs, researchers can use more advanced models, such as GANs and VAEs, that are better able to capture the underlying patterns and distributions of a dataset. Additionally, training on larger and more diverse datasets can also help to improve the quality of generated outputs.
Control Over the Generated Outputs: To have more control over the characteristics of generated outputs, researchers can use techniques such as conditional GANs and VAEs, which allow the model to generate outputs based on a set of specified conditions.
Computational Requirements: To reduce the computational requirements of generative AI systems, researchers can use techniques such as model compression, which can reduce the size and complexity of a model without compromising its performance.
Bias and Fairness: To address bias and fairness, researchers can use techniques such as debiasing and fair representation learning, which can help to remove biases present in the training data. Additionally, researchers can also use techniques such as counterfactual data generation, which can help to generate more diverse and representative training data.
Explainability and Interpretability: To improve the interpretability of generative AI models, researchers can use techniques such as visualization and model interpretability methods, which can help to understand how the model is making its predictions.
Safety and Security: To ensure the safety and security of generative AI systems, researchers can use techniques such as adversarial training and robust optimization, which can help to make the model more resistant to malicious attacks. Additionally, researchers can also use techniques such as watermarking and fingerprinting, which can help establish provenance and trace the origins of generated outputs.
No technology has ever been developed without first addressing the challenges. Generative AI is a rapidly growing technology field with several exciting potential applications. As technology advances, we can expect to see more powerful generative AI systems that can address the limitations and challenges we discussed while also improving upon to create new and unique outputs that are indistinguishable from real-world data.
Generative AI is a class of AI systems that generate new and unique outputs, such as images, text, or music, based on deep learning algorithms. These systems have many potential applications like computer graphics and animation, image and video synthesis, text generation, and music composition. However, there are also some disadvantages, limitations, and challenges to generative AI. These include Quality of generated outputs, control over generated outputs, computational requirements, bias and fairness, explainability and interpretability, and safety and security. These limitations and challenges must be addressed to ensure the effectiveness and safety of generative AI technology.