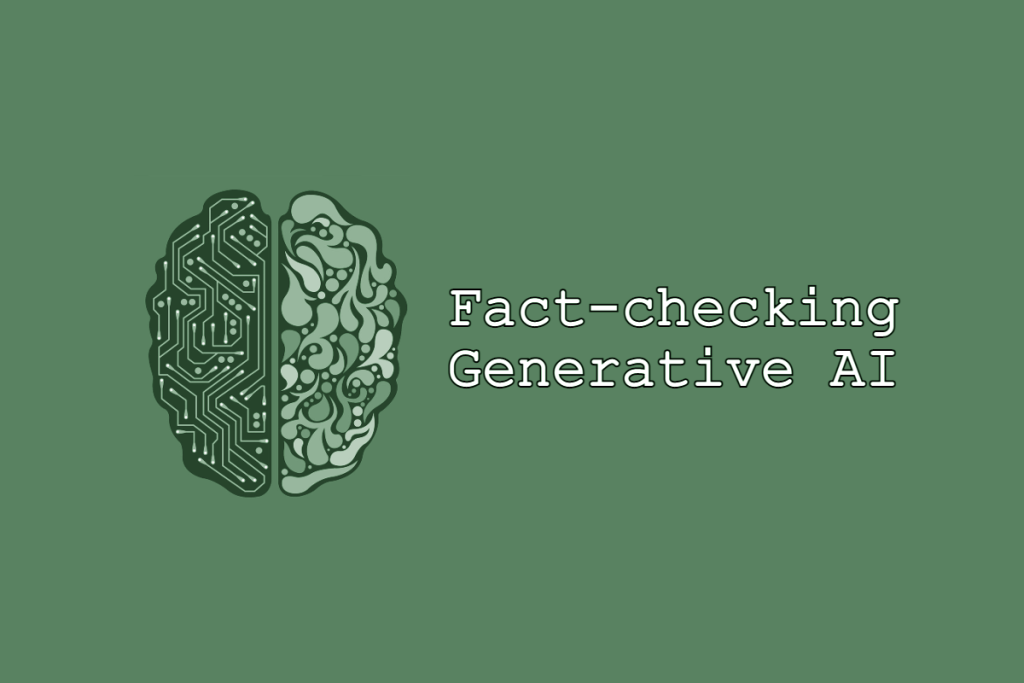
As the technology of Generative AI continues to advance, the ability to create realistic images has become increasingly sophisticated. However, this also means that it has become easier to create and spread false or manipulated images. In this era, it is crucial to have a system in place for image fact-checking to ensure that the images being shared are accurate and not misleading.
Concerns
One of the main concerns with false or manipulated images is the spread of misinformation. These images can be used to spread disinformation and propaganda, potentially causing harm to individuals or groups. Additionally, in an era where social media and the internet have made it easy to share images quickly and widely, the potential for misinformation to spread rapidly is even greater. Image fact-checking can help to combat this by identifying and removing false or manipulated images before they have a chance to spread.
Another concern with false or manipulated images is the potential for them to be used in malicious activities. For example, they can be used to impersonate individuals or organizations, create fake identities, or even to commit crimes. Image fact-checking can help to prevent these types of activities by ensuring that the images being used are legitimate.
Moreover, with the increasing ability to generate realistic images, it will become increasingly difficult to distinguish between real and fake images. This is why image fact-checking is crucial, as it helps to provide a level of authenticity for the images being shared. This can have a positive impact on the trust in images, especially in the field of journalism and news reporting.
Image Fact-checking Methods
Reverse Image Search: This involves using tools such as Google Image Search or TinEye to search for an image online to see if it has been used elsewhere. This can help to determine if an image is original or if it has been manipulated.
Image Metadata Analysis: This involves analyzing the metadata of an image, such as the date and time it was taken, the camera and lens used, and the location where the image was captured. This information can help to determine if an image is authentic or if it has been manipulated.
Human Review: Having a team of trained professionals review images manually is considered a very effective way of image fact-checking. This is particularly useful for images that are not easily identifiable through automated methods.
Image-matching: This technique involves comparing the image with other images from the same event or location. By comparing the images to each other, it can help to identify if an image has been manipulated or not.
Watermark Detection: This technique is used to check if an image has been tampered with by checking for the presence of watermarks, which are added to images to protect them from unauthorized use.
Deep Learning Techniques: With the advancement in deep learning, it is possible to train a model to detect manipulated images. These models can be trained on a large dataset of manipulated and non-manipulated images and can later be used to classify new images as manipulated or not.
It is worth noting that combining these methods can be even more effective than using just one of them, and it’s important to be aware of the limitations of each method and to use them in combination.
Additionally, it is important to keep in mind that as the technology of generative AI continues to advance, it will become increasingly important to stay up-to-date with the latest methods for image fact-checking.
Image Provenance
Establishing the image provenance (the origin or history of an image) can be useful in fact-checking. Knowing the source of an image, where it was taken, and by whom can provide important context and help to determine its authenticity.
For example, if an image is claimed to be from a specific location or event, but the image provenance shows that it was taken elsewhere, this is an indication that the image may have been manipulated or is not original. Additionally, if an image is claimed to be recent, but the image provenance shows that it was taken several years ago, this is also an indication that the image may not be an accurate representation of the present.
Moreover, image provenance can also help to identify the creator or photographer of the image, which can be useful in identifying the credibility of the source. For example, if an image is claimed to be taken by a professional photographer, but the image provenance shows that it was taken by an amateur, this can indicate that the image may not be as credible as it is claimed to be.
Forensics
In addition, image provenance can be useful in digital forensics and investigations, it can help to identify who may have manipulated the image or if it has been tampered with, and can also be used in legal cases if the image is used in a fraudulent or malicious way.
Image Provenance Tools
Provenance tools that can be used to establish the origin and history of an image:
Google Image Search: This allows you to search for an image using a reverse image search, which can help to determine if an image has been used elsewhere and find the original source.
TinEye: A reverse image search tool that can be used to find where an image has been used online, and can also be used to track the image’s history of usage.
FotoForensics: A forensic image analysis tool that can be used to analyze image metadata and find any signs of manipulation.
Mediainfo: A tool that can be used to extract metadata from an image, including information about the camera and lens used, the date and time the image was taken, and more.
ImageTracker: A tool that can be used to track the usage of an image across the internet, including where the image has been used, who has used it, and when.
Veracity.ai: an image-verification platform that uses deep learning algorithms and image-matching to detect manipulated images and also to trace the image’s origin and track its online journey.
ImageIQ: A tool that uses image-matching and deep learning to detect manipulated images and trace the origin of the image.
These are some of the popular tools available, but it is important to keep in mind that new tools and technologies are continually emerging and also some of these tools may have limitations and they could be used in combination with other tools for better results.
Summary
Image fact-checking in the generative AI era involves identifying and removing false or manipulated images to prevent the spread of misinformation and malicious activities. It can be done using methods such as reverse image search, image metadata analysis, human review, image matching, watermark detection, and deep learning-based techniques. Establishing the image provenance, which is the origin and history of an image, is also a valuable step in fact-checking as it can provide important information about the authenticity of the image. Tools such as Google Image Search, TinEye, FotoForensics, Mediainfo, ImageTracker, etc. can be used to establish image provenance.